Exoskeletons Take a Leap Forward with AI
Research published in Nature from X graduate Skip and Georgia Tech demonstrates the potential for AI-powered robotic exoskeletons to help millions of people move more easily
When we began working on our moonshot for mobility at X back in 2019, our team’s goal was to build breakthrough technology to help people who are starting to struggle with movement but don’t need full bodyweight support. According to the CDC, 1 in 4 adults over age 45 face serious difficulty walking or climbing stairs, and reduced mobility often marks the beginning of a person’s decline in physical health and broader quality of life.
Our insight was that if we could find a way to help people move at key moments, like getting up from a chair or walking up stairs, we would be able to support people in staying healthy and active until much later in life. After interviewing hundreds of potential users and experts, we landed on the idea of creating “powered clothing”: A new kind of exoskeleton that used lightweight robotics to provide people with assistance when they needed it and be out of the way when they didn’t.
While exoskeletons have existed for a long time, they tend to be bulky and limited to a few tasks. In order to create an easy to use, everyday product for people, we knew we had to create something hands-free, powered, and, most crucially, adaptable to the varied and unpredictable fluidity of human movement. To help us in this mission, we teamed up with researchers at the Georgia Institute of Technology. Together we explored how machine learning could help us create a wearable exoskeleton that would be fluid and adaptable.
We shared the results of our collaboration in this paper published in Nature. In a global first, we were able to demonstrate that machine learning can be used to continuously and seamlessly assist someone wearing an exoskeleton, regardless of the tasks they’re performing or how quickly their movements change. These results point to the exciting potential for AI and lightweight robotics to help millions of people with early mobility challenges. Read on to learn more about our collaboration and the Nature results.
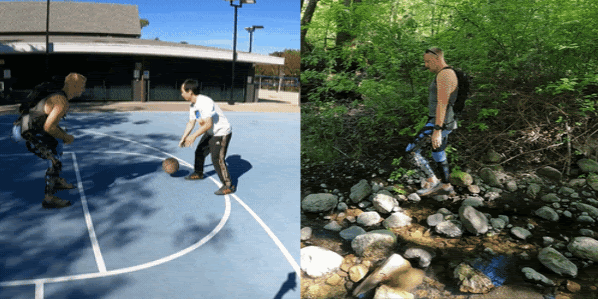
Improving cumbersome exoskeletons with machine learning
The majority of today’s wearable robotics use controllers that are designed to support a small set of predefined movements, such as walking or squatting, and focus on a single joint like the hip, ankle, or knee. These controllers must be optimized, adjusted, and calibrated across activities and people, and when the type of movement changes, the controller must be manually or automatically “switched.” Such limitations make using an exoskeleton a cumbersome, rigid experience. People are limited to performing a standard set of tasks, which fail to account for natural human movement in all its fluid complexity.
While our team was developing our project at the moonshot factory, we decided to explore whether AI and machine learning could help address these limitations. We collected data from early-stage prototypes that we tested across stairs, ramps, walkways, parking lots, and basketball courts in and around X’s headquarters in Mountain View, California. We then used that data to develop machine learning models that could teach exoskeleton controllers to work continuously, dynamically, and for a large range of motions and human bodies.
Along the way, we formed a partnership with Georgia Tech. Their team of researchers had extensive experience with data collection and exoskeletons, but lacked an easy-to-use device for large-scale studies. Our team at X developed and built the exoskeletons the Georgia Tech team used for data collection, and their biomechanical and machine learning expertise helped inform the design of our controller.
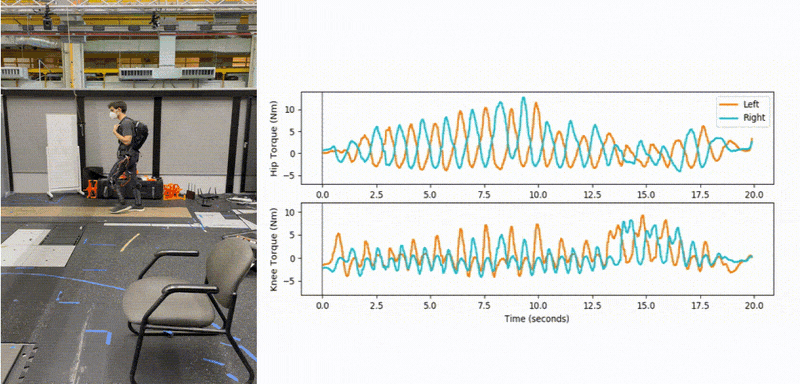
A next-generation ‘wearable robot’
We built our first product as a “wearable robot,” with the functional capabilities of an exoskeleton and the form factor of clothing, designed for supporting an extensive range of movements. Our prototype included actuators on the hip and knee, mounted using carbon fiber and 3D-printed nylon orthotics. We also developed custom pants to integrate the structure tightly with the human body, optimizing for compliance, comfort, and adjustability. On-body sensors at the knee, hip, thigh, shank, and foot were used to monitor and collect joint movement data.
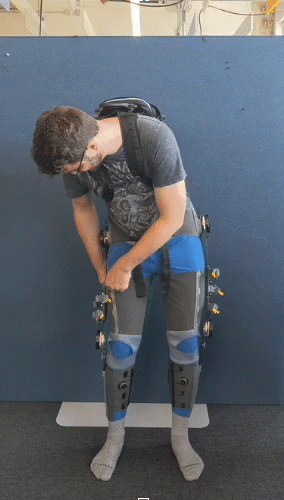
Our prototypes and work in interfaces and controls ultimately led to the breakthrough findings published in Nature. The task-agnostic exoskeleton controller proposed in the paper provides continuous assistance across a range of human motions, without the need for calibration, training, configuring, or manual switching between activities. It can adapt to dynamic movement changes in real-time, basing its functionality on a human’s particular physiological state and allowing for autonomous, coordinated assistance across multiple lower-limb joints at once.
Our machine learning models demonstrated that they could accurately estimate hip and knee joint moments during a broad range of diverse activities, outperforming existing best-in-class methods. People expended less energy, and their joints had to do less work, without any manual controller changes between activities.
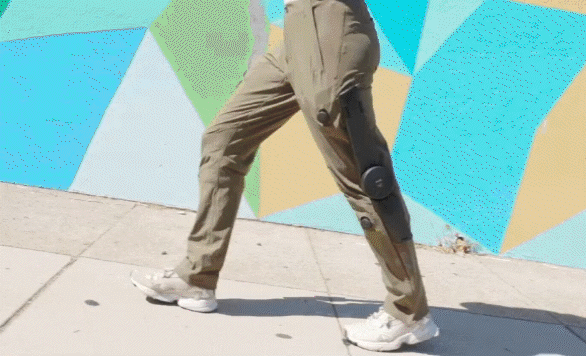
Movewear on the market
After incubating our technology at X, some of our team spun out to form Skip in 2023. Today at Skip, we’re refining the prototypes we developed in collaboration with Georgia Tech to create a new category of powered clothing called “movewear.” Movewear integrates exoskeleton technology into consumer products that support people to move effortlessly, confidently, and joyfully.
Earlier this year, we introduced our first piece of movewear, the MO/GO, in partnership with Arc’Teryx: a pair of powered pants designed to assist with walking, hiking, climbing stairs, and more.
As we continue to design movewear to help with a range of everyday activities, we’re always looking for folks who are interested in collaborating or supporting our journey. Reach out to hello@skipwithjoy.com for more information.
References:
Georgia Tech blogpost: No Matter the Task, This New Exoskeleton AI Controller Can Handle It + Video